The research team led by Professor Gu Yongjian from the Faculty of Information Science and Engineering has published their latest research findings titled Quantum Gated Recurrent Neural Networks in IEEE Transactions on Pattern Analysis and Machine Intelligence (TPAMI), a top-tier journal in computer science and artificial intelligence.
As a research hotspot at the interdisciplinary field of quantum computing and artificial intelligence, quantum neural networks are not only one of the main directions for exploring the advantageous applications of NISQ (Noisy Intermediate-Scale Quantum) computers, but also a significant means to advance the development of artificial intelligence technology. Demonstrating the theoretical and practical advantages of quantum neural networks over traditional classical models is crucial for accelerating the practical application of both quantum computing and artificial intelligence.
“Barren plateau phenomenon” stands as one of the major challenges in quantum neural networks. This phenomenon refers to the tendency of the gradient of the loss function to approach zero across a vast expanse of the parameter space as the quantum neural network scales up, rendering optimization algorithms ineffective in updating parameters and thus leading to a stagnation in optimization. This limitation restricts the scalability of quantum neural networks in addressing complex problems. In response to these challenges, the research team has proposed a novel quantum neural network architecture—the Quantum Gated Recurrent Neural Network (QGRNN). This model integrates the strengths of variational quantum algorithms and classical recurrent networks, establishing a quantum recurrent neural network architecture equipped with a gating mechanism. Significant progress has been achieved both theoretically and in practical applications.
The team has verified the performance advantages of QGRNN through numerous numerical experiments, covering a variety of application scenarios such as long-sequence benchmark problem testing, predictions of gene regulatory network dynamics, and stock market price forecasting. The results demonstrate that QGRNN exhibits significant performance advantages, and holds great potential for application when dealing with long-sequence data and tackling complex dynamical problems.
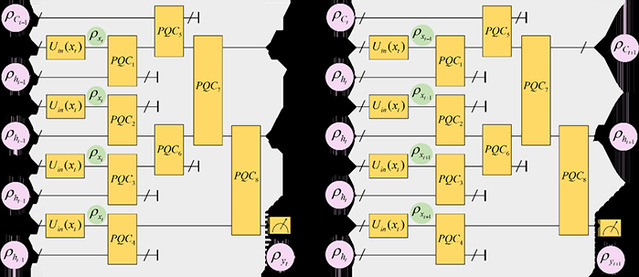
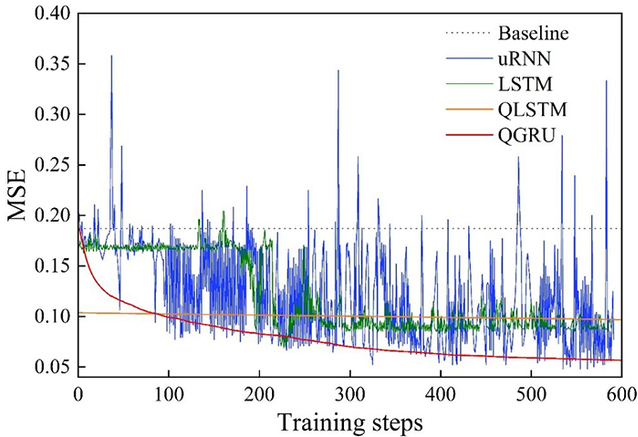
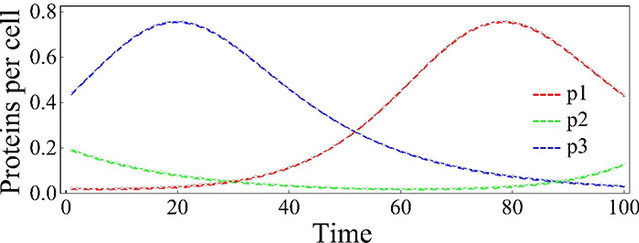
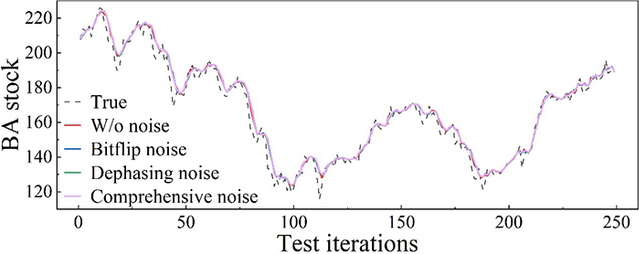